There was a shared understanding
Posted: Wed Dec 18, 2024 3:27 am
At this clinic, customers who came directly to the clinic without making an appointment accounted for approximately 80% of all new visits, meaning that CV (conversion) data for approximately 80% of the total was not being measured .
For this reason, with the client that manually phone number details usa adjusting bid prices based on the operator's experience would be more likely to lead to results than optimizing using automatic bidding, and so they had been operating with manual bidding for some time.
Additionally, to control the delivery volume for each store, campaigns were previously run separately for each of the more than 50 stores nationwide. Although this was able to achieve the desired advertising effect, in order to increase advertising effectiveness more efficiently, it was decided to make fundamental reforms to advertising operations, including campaign design and bidding strategies. In other words, in this case, in addition to switching to automatic bidding, the account structure was reviewed and restructured from the ground up.
Please see the following article for points to consider when reviewing your account structure if you are using automated bidding.
A case study of how reviewing account structure improved search advertising results
It is becoming increasingly important to utilize machine learning in search advertising. Especially when using automated bidding, it is essential to optimize your account structure for machine learning in order to improve your results...
3. Hypothesis for the problem
First of all, from previous discussions with clients and experience with data analysis, we knew the following:
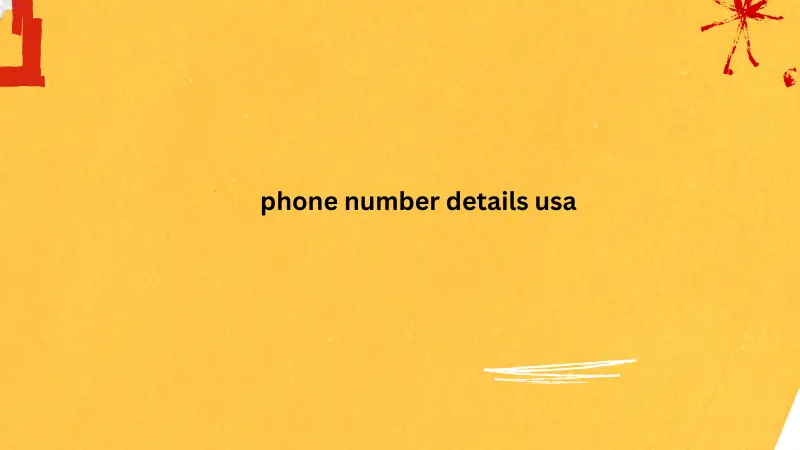
Since this is a product with low impulsivity and a long consideration period, and there should be a lot of online behavior history, the introduction of automatic bidding is expected to improve WebCV.
Although there is a yield, both users who make a reservation in advance online and those who visit the store directly without a reservation take action, so it is likely that there is no significant difference in user behavior on the web.
When the client shared with us "interview data of users who visited the clinic," we found that even the same user's conversion points were different, with some making advance reservations online and others coming to the clinic without a reservation.
Based on the above facts, we hypothesized that there would be no significant differences in demographic attributes or online user behavior between users who convert online and those who visit the store in person. We determined that there would be no major problems in this test if we did not use offline data from users who visit the store in person without an appointment as learning material, and therefore decided to operate automatic bidding using only WebCVs as learning material .
Additionally, while previously campaign designs were separate for each store, the introduction of automated bidding required securing a certain amount of learning data (web conversion data) to further optimize machine learning, so we felt that we needed to reconsider our campaign designs.
4. Details of the measures implemented
In conjunction with the introduction of automatic bidding, we took the following measures:
The main verification medium is listing ads + (P-MAX)
In order to secure the amount of learning data, some regional campaigns were consolidated, and operations were integrated from store-by-store into campaigns for prefectures such as "Tokyo," "Chiba," and "Saitama."
In order to compare results by region and verify effectiveness, automatic bidding was applied only to campaigns in the metropolitan areas of "Tokyo," "Chiba," "Saitama," and "Kanagawa," while manual bidding was continued for campaigns by store in other regions.
Reference: Estimated conversion data required for optimizing machine learning for major media
In reality, it is not necessary to meet the following criteria as it depends on the industry and the purpose of the account, but these are the recommended number of conversions generally required when using automatic bidding for each media.
Google Ads:
・When operating with target return on advertising spend (tROAS), 15 or more conversions are recommended in the past 30 days on a campaign basis.
・There are no media recommendations for other automatic bidding strategies, but from experience, when using target cost per acquisition (tCPA), machine learning is unlikely to work well unless 30 or more conversions are obtained in the past 30 days.
Yahoo! Ads:
・When using target cost per acquisition (tCPA), a minimum of 30 or more conversions are recommended in the past 30 days.
・When using target return on investment (tROAS), a minimum of 50 or more conversions are recommended in the past 30 days.
Meta Ads:
・A minimum of 50 conversions per week are recommended per ad set (a minimum of 50 or more conversions per week per campaign if optimizing with campaign budget)
*Excerpt from media company materials
For this reason, with the client that manually phone number details usa adjusting bid prices based on the operator's experience would be more likely to lead to results than optimizing using automatic bidding, and so they had been operating with manual bidding for some time.
Additionally, to control the delivery volume for each store, campaigns were previously run separately for each of the more than 50 stores nationwide. Although this was able to achieve the desired advertising effect, in order to increase advertising effectiveness more efficiently, it was decided to make fundamental reforms to advertising operations, including campaign design and bidding strategies. In other words, in this case, in addition to switching to automatic bidding, the account structure was reviewed and restructured from the ground up.
Please see the following article for points to consider when reviewing your account structure if you are using automated bidding.
A case study of how reviewing account structure improved search advertising results
It is becoming increasingly important to utilize machine learning in search advertising. Especially when using automated bidding, it is essential to optimize your account structure for machine learning in order to improve your results...
3. Hypothesis for the problem
First of all, from previous discussions with clients and experience with data analysis, we knew the following:
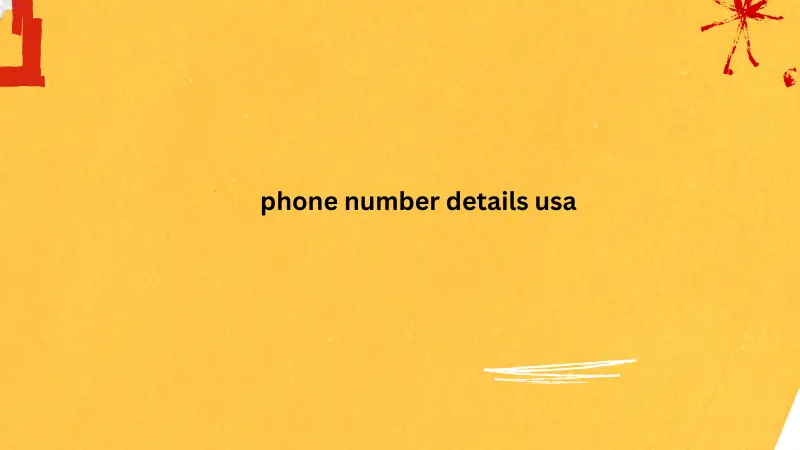
Since this is a product with low impulsivity and a long consideration period, and there should be a lot of online behavior history, the introduction of automatic bidding is expected to improve WebCV.
Although there is a yield, both users who make a reservation in advance online and those who visit the store directly without a reservation take action, so it is likely that there is no significant difference in user behavior on the web.
When the client shared with us "interview data of users who visited the clinic," we found that even the same user's conversion points were different, with some making advance reservations online and others coming to the clinic without a reservation.
Based on the above facts, we hypothesized that there would be no significant differences in demographic attributes or online user behavior between users who convert online and those who visit the store in person. We determined that there would be no major problems in this test if we did not use offline data from users who visit the store in person without an appointment as learning material, and therefore decided to operate automatic bidding using only WebCVs as learning material .
Additionally, while previously campaign designs were separate for each store, the introduction of automated bidding required securing a certain amount of learning data (web conversion data) to further optimize machine learning, so we felt that we needed to reconsider our campaign designs.
4. Details of the measures implemented
In conjunction with the introduction of automatic bidding, we took the following measures:
The main verification medium is listing ads + (P-MAX)
In order to secure the amount of learning data, some regional campaigns were consolidated, and operations were integrated from store-by-store into campaigns for prefectures such as "Tokyo," "Chiba," and "Saitama."
In order to compare results by region and verify effectiveness, automatic bidding was applied only to campaigns in the metropolitan areas of "Tokyo," "Chiba," "Saitama," and "Kanagawa," while manual bidding was continued for campaigns by store in other regions.
Reference: Estimated conversion data required for optimizing machine learning for major media
In reality, it is not necessary to meet the following criteria as it depends on the industry and the purpose of the account, but these are the recommended number of conversions generally required when using automatic bidding for each media.
Google Ads:
・When operating with target return on advertising spend (tROAS), 15 or more conversions are recommended in the past 30 days on a campaign basis.
・There are no media recommendations for other automatic bidding strategies, but from experience, when using target cost per acquisition (tCPA), machine learning is unlikely to work well unless 30 or more conversions are obtained in the past 30 days.
Yahoo! Ads:
・When using target cost per acquisition (tCPA), a minimum of 30 or more conversions are recommended in the past 30 days.
・When using target return on investment (tROAS), a minimum of 50 or more conversions are recommended in the past 30 days.
Meta Ads:
・A minimum of 50 conversions per week are recommended per ad set (a minimum of 50 or more conversions per week per campaign if optimizing with campaign budget)
*Excerpt from media company materials